Dr. Milena Rabovsky
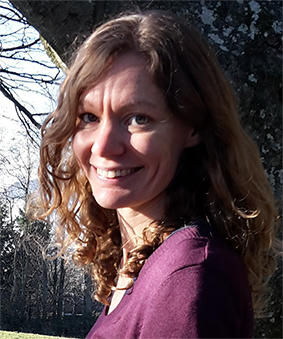
Please visit my current website for the latest information about my research.
Language ultimately aims to convey meaning. Yet, the processing of meaning remains elusive and much less understood as compared to other aspects of language such as the processing of syntax, orthography, or phonology. The goal of my research is to elucidate the processing of meaning in language, relying on the following guiding principles: First, to understand human language comprehension, we need to take the evidence provided by neuroscientific data – such as event-related brain potentials (ERPs) providing direct online indicators of electrical brain activity during comprehension – seriously, even at the cost of trading longheld beliefs about how language comprehension should work in principle. Second, it is crucial to precisely understand what these brain signals mean in terms of underlying processes. A principled way to understand a process is to rebuild it and therefore an important complementary approach I use, besides empirical EEG experiments (Rabovsky et al., 2008, 2012a, 2012b, 2012c, 2019), is the simulation of language-related brain signals with computationally explicit and theoretically precise implemented neural network models.
Specifically, the most widely used ERP component in research on language and meaning is the N400 component. Since the first report of larger N400 amplitudes in sentences with semantic incongruities such as “I take my coffee with cream and dog.”, N400 amplitudes have been found to be modulated by numerous lexical and semantic variables. However, despite more than 30 years of research and over 1000 empirical studies, the functional basis of N400 amplitudes remains unclear and actively debated (Kutas & Federmeier, 2011). To better understand the functional basis of N400 amplitudes, we simulated a broad range of empirically observed N400 effects with implemented neural network models. Results suggest that N400 amplitudes reflect the stimulus-induced change in an implicit and probabilistic representation of meaning, and that this change at the same time corresponds to the implicit prediction error contained in the previous representation (Rabovsky, Hansen, & McClelland, 2018; Rabovsky & McRae, 2014). It is often assumed that prediction errors drive learning so that our simulations suggest that larger N400 amplitudes should trigger enhanced adaptation. This model-derived prediction is currently tested in empirical EEG experiments.